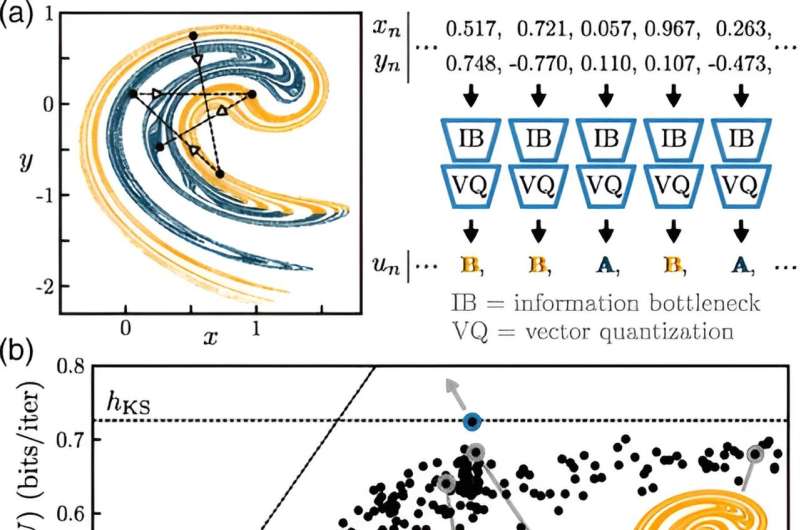
How do we measure chaos and why would we want to? Together, Penn engineers Dani S. Bassett, J. Peter Skirkanich Professor in Bioengineering and in Electrical and Systems Engineering, and postdoctoral researcher Kieran Murphy leverage the power of machine learning to better understand chaotic systems, opening doors for new information analyses in both theoretical modeling and real-world scenarios.
Humans have been trying to understand and predict chaotic systems such as weather patterns, the movement of planets and population ecology for thousands of years. While our models have continued to improve over time, there will always remain a barrier to perfect prediction.
That’s because these systems are inherently chaotic. Not in the sense that blue skies and sunshine can turn into thunderstorms and torrential downpours in a second, although that does happen, but in the sense that mathematically, weather patterns and other chaotic systems are governed by physics with nonlinear characteristics.
“This nonlinearity is fundamental to chaotic systems,” says Murphy. “Unlike linear systems, where the information you start with to predict what will happen at timepoints in the future stays consistent over time, information in nonlinear systems can be both lost and generated through time.”
Like a game of telephone where information from the original source gets lost as it travels from person to person while new words and phrases are added to fill in the blanks, outcomes in chaotic systems become harder to predict as time passes. This information delay thwarts our best efforts to accurately forecast the weather more than a few days out.
“You could put millions of probes in the atmosphere to measure wind speed, temperature and precipitation, but you cannot measure every single atom in the system,” says Murphy.
“You must have some amount of uncertainty, which will then grow, and grow quickly. So while a prediction for the weather in a few hours might be fairly accurate, that growth in uncertainty over time makes it impossible to predict the weather a month from now.”
In their recent paper published in Physical Review Letters, Murphy and Bassett applied machine learning to classic models of chaos, physicists’ reproductions of chaotic systems that do not contain any external noise or modeling imperfections, to design a near-perfect measurement of chaotic systems to one day improve our understanding of systems including weather patterns.
“These controlled systems are testbeds for our experiments,” says Murphy.
“They allow us to compare with theoretical predictions and carefully evaluate our method before moving to real-world systems where things are messy and much less is known. Eventually, our goal is to make ‘information maps’ of real-world systems, indicating where information is created and identifying what pieces of information in a sea of seemingly random data are important.”
Previous studies have used other kinds of measurements to describe information creation in chaotic systems, such as with Lyapunov exponents, which describe the exponential divergence or convergence of points moving in space and time. However, this approach requires either knowledge of the governing equations in a particular system or else massive amounts of data. By leveraging machine learning, a new approach can describe information creation in chaotic systems with a lot less data.
Machine learning has traditionally been used to identify patterns in large data sets and then make predictions as to how that data will behave through time, across locations or any other variable in question. In Murphy’s study of chaos, he is not using machine learning to make predictions; rather, he is using machine learning to encapsulate an entire chaotic system and boil it down to one measurement.
“Our measurement is informed by deep learning, an algorithm extracting information from all of the states of the system at every time point,” he says. “We are able to take these complex systems and distill the essence of their dynamics in cases that would be too complicated for our human intuition to tease apart and make sense of.”
By keeping as much important information and discarding as much unimportant information as possible, the resulting measurement gets closer to a perfect measurement of chaotic systems, a step closer to designing better measurements for weather forecasting and to understanding fundamental barriers to prediction.
In his work, Murphy uses machine learning to do multivariate information theory, meaning he is looking to understand systems with multiple sources of information (which is true for almost all systems). He has generally examined these systems at one time point, as in a scenario where a patient comes into a doctor’s office and has many different tests done to determine their health. But now, with this work on chaos, Murphy is developing methods to understand systems across time, where time becomes another variable in this multivariate perspective.
“With each system we study, we’re building out a powerful framework for mapping out the information in data, made possible by the unique strengths of machine learning,” he says. “I am continually amazed by the depth of insight we can pull out of data, and I’m excited for the new directions opened up by this chaos project for studying the dynamics of complex systems.”
Murphy and Bassett’s work on chaos has also given rise to the investigation of dynamic systems in the human body.
“The ability to understand information creation in complex dynamical systems is now motivating new approaches to one of the lab’s core questions: How does the brain enable the mind?,” says Bassett. “Deciphering the information creation in the human brain holds notable promise for mental health and well-being.”
While complete certainty is not possible, humans have an innate desire to pursue it. Advancing our ability to predict and understand these dynamic systems not only helps us get closer to that certainty, the pursuit and the new tools we integrate into it can help us find out-of-the-box interpretations of patterns and data to inform innovative solutions to real-world problems from weather forecasting to medical diagnostics and the improvement of our overall quality of life.
More information:
Kieran A. Murphy et al, Machine-Learning Optimized Measurements of Chaotic Dynamical Systems via the Information Bottleneck, Physical Review Letters (2024). DOI: 10.1103/PhysRevLett.132.197201
Provided by
University of Pennsylvania
Citation:
Engineers use machine learning to measure chaos in systems (2024, July 30)
retrieved 30 July 2024
from https://phys.org/news/2024-07-machine-chaos.html
This document is subject to copyright. Apart from any fair dealing for the purpose of private study or research, no
part may be reproduced without the written permission. The content is provided for information purposes only.