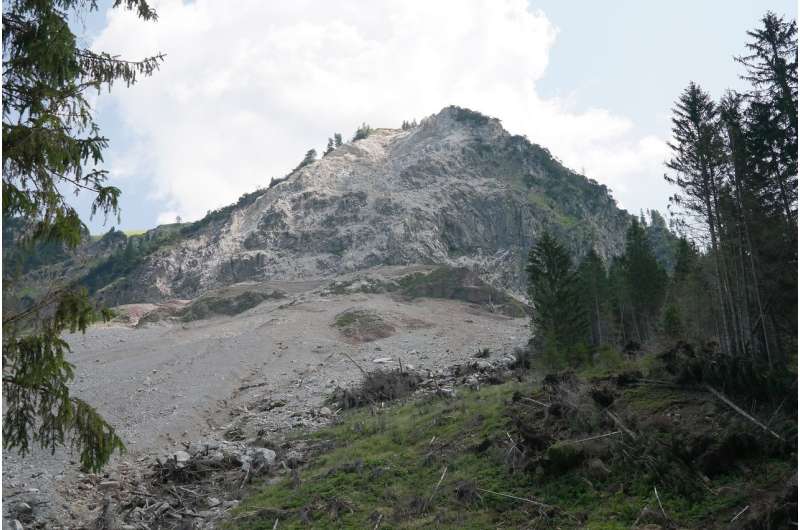
Using data collected from a 2022 magnitude 6.8 earthquake in Luding County in China’s Sichuan Province, researchers have tested whether Global Navigation Satellite System (GNSS) observations could be used for rapid prediction of earthquake-triggered landslides.
In their report in Seismological Research Letters, Kejie Chen of the Southern University of Science and Technology and colleagues share a set of methods for near real-time GNSS landslide prediction. Some of their models accurately identified about 80% of the landslide locations that were triggered by the Luding earthquake, the researchers found.
Based on their results, Chen and colleagues show that near real-time landslide prediction for an earthquake like the Luding event could be completed in approximately 40 minutes—a time that is likely to be improved with further development of their models and higher-speed computing, they noted.
The 5 September 2022 Luding earthquake on the southeastern segment of the Xianshuihe Fault led to more than 6,000 landslides, which caused severe damage to over 3,500 square kilometers of the region.
“The number of co-seismic landslides triggered by the Luding earthquake was significant but not entirely unexpected given the region’s topography and seismic activity. The area is known for its susceptibility to landslides, especially following large seismic events,” said Chen. “However, the scale of destruction and the specific locations affected did provide new insights into the region’s risk profile and highlighted the importance of continuous monitoring and improved prediction models.”
GNSS data measures the movement of the ground during an earthquake. Chen and colleagues had already been exploring the use of GNSS data for locating earthquake sources and tsunami early warning when the Luding earthquake struck.
“For earthquakes that rupture inland, especially in mountainous areas in China, landslides become the main cascading seismic hazard,” Chen explained. “Our research has been focused on developing and refining methods for landslide prediction using GNSS. The Luding earthquake provided a critical case study that allowed us to evaluate and adapt our methods in the context of co-seismic landslides.”
The researchers developed an end-to-end GNSS prediction method, which begins by constructing slip models of the event based on the GNSS offset and displacement waveform data. They then used physics-based simulations of the earthquake with those slip models to obtain a measurement of peak ground velocity.
Finally, Chen and colleagues used the peak ground velocity with a machine learning algorithm to predict a possible spatial distribution of landslides for the event. Six Chinese earthquakes ranging in magnitude from 6.1 to 8.0 that shared geological similarities with the Luding earthquake were used to train the prediction algorithm.
One way to enhance the method would be to combine GNSS observations with data on near-fault ground motion waveforms captured by low-cost accelerometers called MEMS, Chen and colleagues noted. To improve earthquake warning and response, China has recently included more than 10,000 MEMS-based stations in a nationwide earthquake warning system.
“Using both data types in a complementary manner enhances the robustness and accuracy of landslide prediction,” Chen said. “GNSS data can validate and refine the predictions made by MEMS data, ensuring a comprehensive monitoring system.”
More information:
Lei Xia et al, Feasibility of Coseismic Landslide Prediction Based on GNSS Observations: A Case Study of the 2022 Ms 6.8 Luding, China, Earthquake, Seismological Research Letters (2024). DOI: 10.1785/0220240069
Provided by
Seismological Society of America
Citation:
Sichuan Province earthquake offers lessons for landslide prediction from GNSS observations (2024, August 7)
retrieved 7 August 2024
from https://phys.org/news/2024-08-sichuan-province-earthquake-lessons-landslide.html
This document is subject to copyright. Apart from any fair dealing for the purpose of private study or research, no
part may be reproduced without the written permission. The content is provided for information purposes only.